Artificial Intelligence (AI) has transformed how enterprises operate, enabling automation, data-driven decision-making, and innovative solutions across industries. However, with this transformation comes the responsibility to govern AI effectively. Enterprise AI governance refers to the frameworks, policies, and practices organizations adopt to ensure that their AI systems align with ethical principles, legal standards, and organizational goals.
The stakes are high for enterprises utilizing AI. Ethical missteps, such as biased algorithms or privacy violations, can lead to reputational damage, financial losses, and legal penalties. As regulatory bodies worldwide introduce stringent AI laws, businesses must proactively address these challenges to mitigate risks and maintain trust.
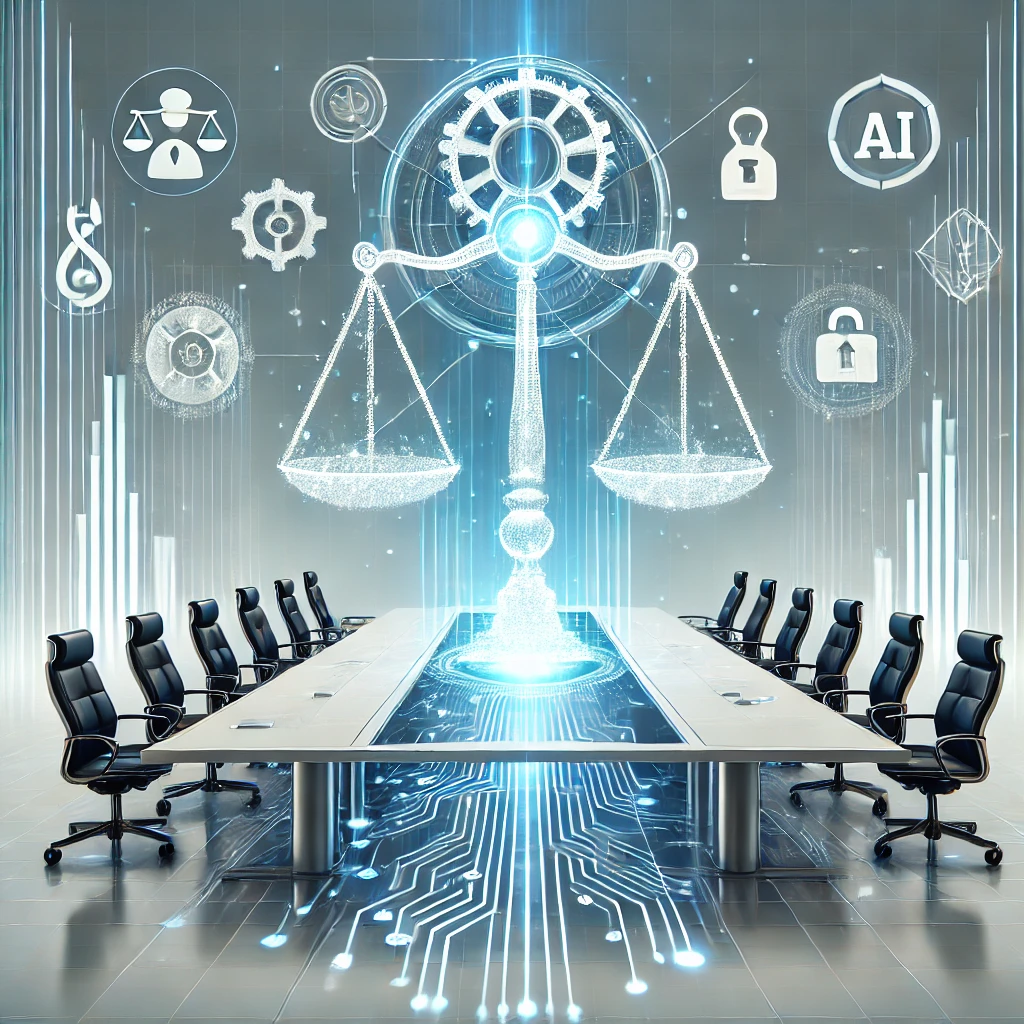
1. The Landscape of AI in Enterprises
Artificial Intelligence (AI) has swiftly transitioned from being a futuristic concept to a cornerstone of modern enterprises. It has reshaped industries by enabling automation, data-driven decision-making, and innovation. However, while AI offers immense opportunities, it also brings complex challenges that businesses must navigate to ensure sustainable growth and ethical practices.
Understanding AI in Enterprises
AI has become a cornerstone of modern enterprises, reshaping operations through automation, predictive analytics, and customer engagement tools. From retail to healthcare, AI-driven solutions are delivering substantial value.
Adoption Trends and Opportunities
AI adoption rates are climbing across sectors, with enterprises leveraging AI to reduce costs, improve decision-making, and innovate. A Gartner report highlights that 91% of enterprises are actively investing in AI technologies.
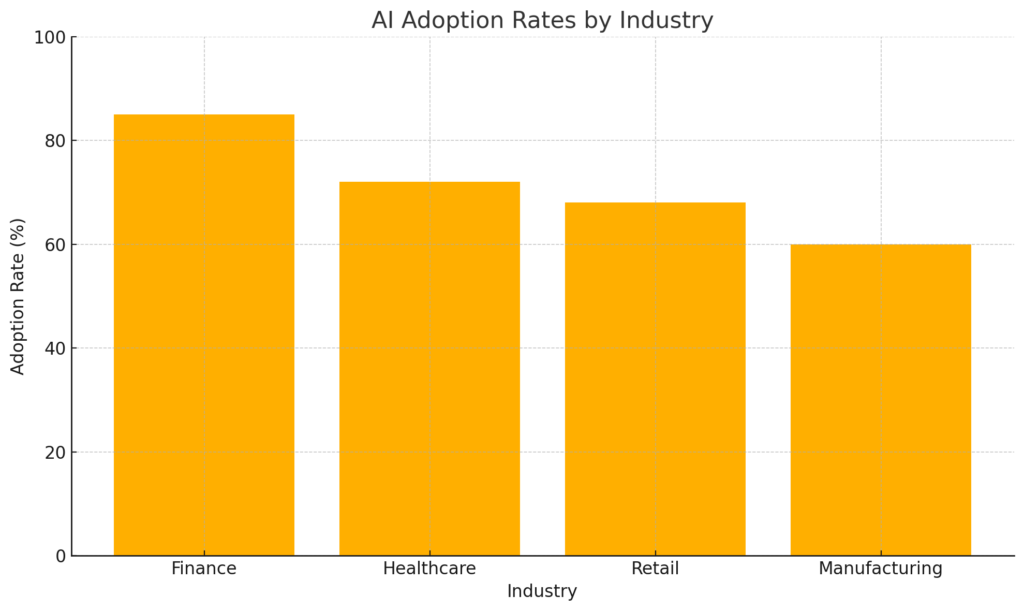
Industry | AI Adoption Rate | Key Applications |
---|---|---|
Finance | 85% | Fraud detection, risk analysis |
Healthcare | 72% | Diagnostics, personalized medicine |
Retail | 68% | Recommendation systems, demand planning |
Manufacturing | 60% | Predictive maintenance, quality control |
Associated Risks and Challenges
While the benefits of AI are substantial, enterprises face significant risks. Challenges include biases in algorithms, lack of explainability, data privacy issues, and accountability for AI-driven decisions.
2. Ethical Challenges in AI Implementation
As AI becomes more pervasive, enterprises must address ethical dilemmas to maintain trust and avoid unintended consequences. Ethical AI implementation is not just about compliance but about building systems that reflect organizational values and societal norms.
Bias and Discrimination
Bias in AI can result from skewed training datasets, leading to discriminatory outcomes. For instance, tools like IBM’s AI Fairness 360 can help identify and mitigate bias by providing comprehensive analysis and remediation techniques, ensuring more equitable AI systems.
Additionally, regularly auditing algorithms and incorporating diverse datasets during training are essential steps. For example, an AI recruiting tool may favour certain demographics due to historical data patterns.
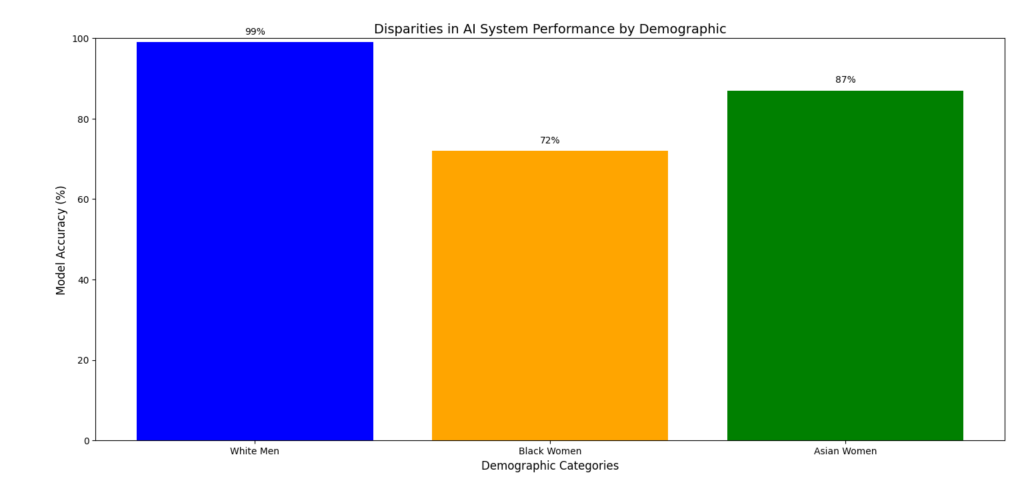
Discrimination Statistics:
- Studies show that facial recognition systems are 99% accurate for White men but drop to 65-75% accuracy for Black women (source: MIT Media Lab).
- Amazon’s AI recruiting tool was scrapped after it was found to favor male candidates over female candidates due to biased training data.
Real-World Examples:
- COMPAS Algorithm: Showed racial bias in predicting recidivism rates for Black defendants.
- Google Photos Incident: Labeled Black individuals as “gorillas” due to poor training data diversity.
- Credit Approval AI: Apple Card reportedly provided significantly higher credit limits to men than women with the same financial background.
Mitigation Strategies:
- Use diverse datasets during training.
- Conduct algorithm audits to uncover and address biases.
- Employ AI auditing and AI Governance tools to assess and mitigate bias.
Transparency and Explainability
AI systems often operate as “black boxes,” where the logic behind decisions is not easily understood. This lack of transparency can erode stakeholder trust.
Solutions:
- Implement Explainable AI (XAI) techniques to clarify decision-making processes.
- Use models such as SHAP or LIME for interpretability.
Privacy Concerns
AI systems handle large amounts of sensitive data, making privacy a top concern. Enterprises must comply with stringent data protection regulations like GDPR and CCPA.
Best Practices:
- Anonymize and encrypt personal data.
- Implement strong data governance policies.
Accountability and Liability
Assigning responsibility for AI outcomes is a complex but necessary task. Clear accountability structures and governance frameworks help address this issue.
3. Navigating Regulatory Compliance
Global AI regulations are evolving rapidly, with governments enacting laws to ensure responsible AI use. Enterprises must stay informed and adapt to meet these requirements.
Overview of Global AI Regulations
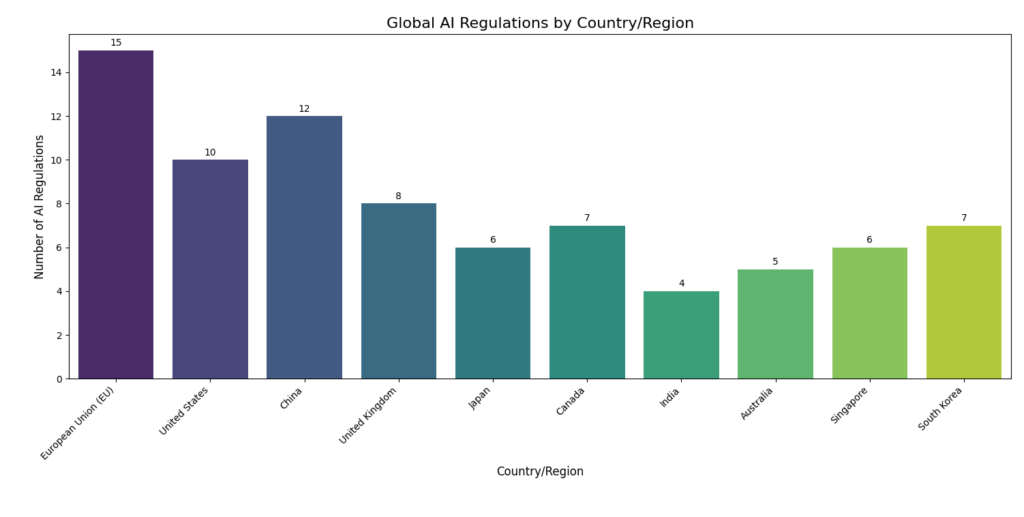
Country/Region | Number of AI Regulations | Notable AI Regulatory Frameworks |
---|---|---|
European Union (EU) | 15 | EU AI Act, GDPR, Digital Services Act |
United States | 10 | Algorithmic Accountability Act, Executive Orders |
China | 12 | AI Governance Regulations, Data Security Law |
United Kingdom | 8 | National AI Strategy, Online Safety Bill |
Japan | 6 | AI Governance Guidelines, Society 5.0 Policies |
Canada | 7 | AIDA (Artificial Intelligence and Data Act), Privacy Legislation |
India | 4 | NITI Aayog AI Strategy, IT Act |
Australia | 5 | AI Ethics Framework, Online Safety Act |
Singapore | 6 | Model AI Governance Framework, PDPA |
South Korea | 7 | AI Development Promotion Act, Digital Framework Act |
Key regulatory frameworks include:
1. EU Artificial Intelligence Act
Focus: Risk-based regulation, transparency, and accountability.
Details:
- The EU AI Act is one of the most comprehensive regulatory frameworks globally. It classifies AI systems into four risk categories:
- Unacceptable Risk: Bans systems like social scoring and subliminal manipulation.
- High Risk: Requires strict assessments (e.g., in healthcare, law enforcement).
- Limited Risk: Includes transparency obligations (e.g., chatbots must disclose they are AI).
- Minimal or No Risk: Encourages innovation without heavy regulation.
- The Act also mandates transparency in AI usage, robust documentation, and compliance assessments for high-risk AI systems.
- Penalties for violations can reach up to 6% of a company’s global annual revenue.
2. U.S. AI Bill of Rights
Focus: Advocates for equity, privacy, accountability, and individual protections.
Details:
- This initiative by the White House is a non-binding framework providing guiding principles for ethical AI development and deployment.
- The five core principles include:
- Safe and Effective Systems: Ensures rigorous testing for safety and usability.
- Protection from Algorithmic Discrimination: Promotes equity by preventing AI bias.
- Data Privacy: Safeguards individuals’ data through secure practices.
- Notice and Explanation: Users should know when AI is being used and how decisions are made.
- Human Alternatives: Ensures that critical decisions (e.g., credit, healthcare) provide human oversight or alternatives.
- While the document sets ethical benchmarks, it lacks enforcement mechanisms, leaving actual regulatory action to states or sector-specific bodies.
3. China’s AI Guidelines
Focus: Cybersecurity, data protection, and ethical AI usage.
Details:
- China’s AI regulations emphasize state control, alignment with national priorities, and cybersecurity compliance.
- The Measures for Generative AI Services (effective August 2023) include:
- AI developers must align their systems with “socialist core values.”
- Mandatory data review for training AI systems, with fines for non-compliance.
- Clear labeling of AI-generated content to prevent misinformation.
- Broader policies like the Data Security Law and Personal Information Protection Law govern data collection, cross-border data transfer, and cybersecurity for AI systems.
- China’s proactive stance also includes heavy investments in AI R&D to dominate global innovation.
Region | Key Regulation | Focus Area |
---|---|---|
EU | Artificial Intelligence Act | Risk management, transparency |
USA | Blueprint for AI Bill of Rights | Privacy, equity, accountability |
China | AI Ethics Guidelines | Cybersecurity, data sovereignty |
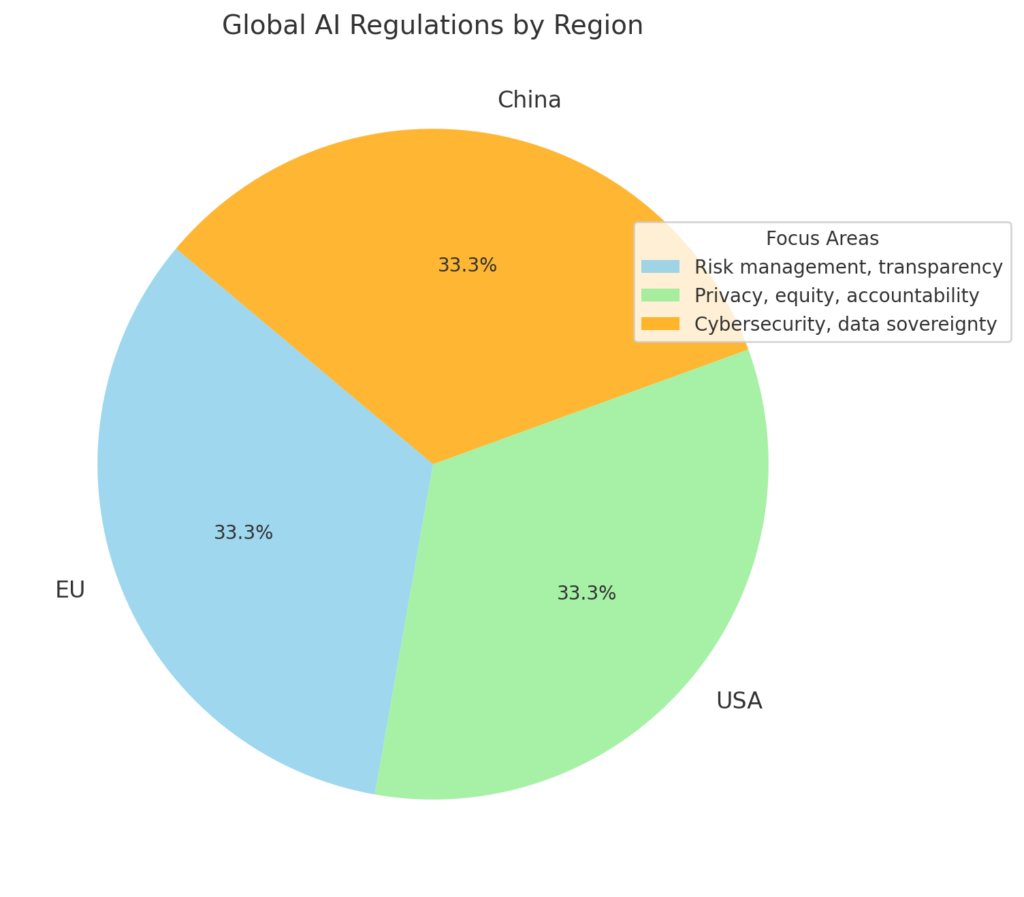
Industry-Specific Standards
Each industry faces unique challenges:
- Healthcare: HIPAA compliance for patient data.
- Finance: Adherence to anti-money laundering (AML) guidelines.
- Automotive: Ensuring safety in autonomous systems.
Data Protection Laws
Laws like GDPR and CCPA mandate robust data handling and privacy measures for AI applications, significantly influencing how industries structure their AI governance frameworks. For instance, in healthcare, compliance with GDPR ensures stringent protection of patient data, while in finance, adherence to CCPA enhances transparency in customer data usage and reinforces trust. Enterprises must integrate these principles into their governance frameworks.
4. Strategies for Effective AI Governance- Building a Robust Framework
Developing a robust AI governance framework is essential for ethical and compliant AI deployment. This involves setting clear guidelines, assigning responsibilities, and implementing accountability mechanisms.
Establishing AI Ethics Committees
Cross-functional AI ethics committees can oversee the governance process. These teams include members from legal, technical, and ethical domains.
Developing Ethical AI Frameworks
Frameworks like Microsoft’s Responsible AI Standard provide actionable guidelines to ensure AI systems align with ethical principles.
Implementing Bias Mitigation Techniques
Bias can be reduced through:
- Regular audits.
- Diverse training datasets.
- Counterfactual testing to simulate alternative scenarios.
Ensuring Transparency and Explainability
Transparency builds trust. Explainable AI techniques make it easier for stakeholders to understand how decisions are made, fostering confidence in AI systems.
5. Ensuring Regulatory Compliance
Regular compliance checks and proactive measures ensure that enterprises meet evolving regulatory standards.
Regular Compliance Audits
Frequent audits help identify gaps and align systems with legal frameworks. LLMOps platforms, for example, can streamline compliance monitoring.
Data Governance Policies
A strong focus on data security and quality ensures that AI systems operate within regulatory boundaries.
Employee Training Programs
Training employees on AI compliance and ethics builds a culture of responsibility and accountability.
Collaboration with Legal Experts
Consulting with legal professionals ensures enterprises are well-prepared to navigate complex regulatory environments.
6. Case Studies
Examining both successes and failures in AI governance offers valuable insights for enterprises.
Successful Implementations
- Google: Developed explainable AI tools to enhance transparency in healthcare diagnostics.
- JP Morgan: Adopted an AI governance framework to address compliance in financial transactions.
Lessons from Failures
- Amazon: An AI recruiting tool was abandoned due to gender bias, illustrating the importance of robust bias mitigation strategies.
AI Ethical Failures Data Table:
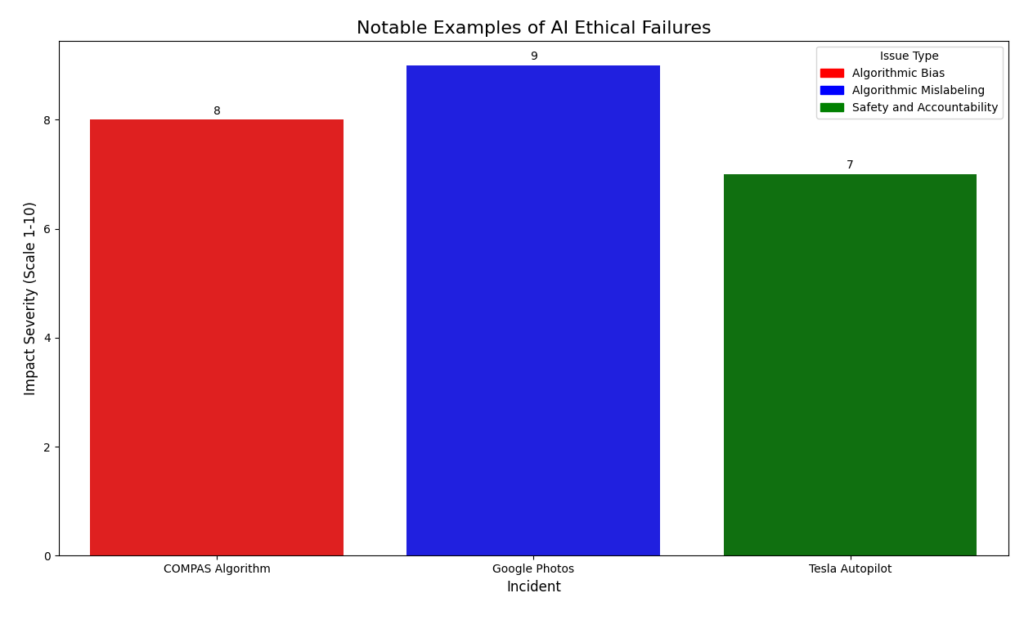
Incident | Issue Type | Description | Impact |
---|---|---|---|
COMPAS Algorithm | Algorithmic Bias | Predicted higher recidivism rates for Black defendants compared to White defendants. | Led to concerns about fairness in criminal justice and calls for transparency in algorithms. |
Google Photos | Algorithmic Mislabeling | Labeled images of Black individuals as “gorillas,” highlighting a failure in training data. | Public outrage and significant reputational damage to Google. |
Tesla Autopilot | Safety and Accountability | Accidents involving self-driving cars due to overreliance and unclear liability standards. | Raised concerns about AI’s reliability and safety in critical applications. |
7. The Road Ahead for AI Governance
As AI technologies evolve, so too will the challenges and opportunities they present.
Evolving Regulations
Regulatory bodies are likely to introduce stricter requirements, emphasizing accountability and transparency.
Technological Advancements
Emerging technologies like Retrieval-Augmented Generation (RAG) models promise to enhance explainability and reduce operational costs.
Continuous Improvement
Ongoing evaluation and adaptation of governance frameworks will be critical to addressing new ethical and regulatory challenges.
Conclusion
- Effective AI governance ensures ethical, transparent, and compliant AI systems.
- Addressing ethical challenges like bias and privacy is crucial for building trust.
- Enterprises must proactively adapt to evolving regulations and leverage advanced tools for governance.
Sources:
https://www.spglobal.com/en/research-insights/special-reports/the-ai-governance-challenge
https://link.springer.com/article/10.1007/s43681-022-00143-x
https://www.gartner.com/en/articles/ai-ethics
https://www.ibm.com/thought-leadership/institute-business-value/en-us/report/ai-governance