Artificial Intelligence (AI) is reshaping industries with its transformative capabilities, yet it brings complex ethical challenges, including concerns around privacy, bias, transparency, and accountability. To ensure AI contributes positively to society, it’s vital for decision-makers—particularly in AI governance, AI compliance, and technology sectors—to integrate responsible practices into AI development.
This article will explore core ethical principles such as fairness, transparency, privacy, and accountability, providing actionable strategies for implementation. We’ll also examine global initiatives, including the EU’s AI Act, that shape standards for ethical AI, equipping readers with insights to balance innovation with responsibility.
Key Ethical Principles in AI Development
For AI to serve society responsibly, it must align with foundational ethical principles. These principles ensure AI applications are fair, transparent, private, and accountable, mitigating risks and fostering trust among users. This section explores key principles that organizations can implement to address ethical concerns in AI, starting with fairness and bias mitigation.
Fairness and Bias Mitigation
Ensuring fairness in AI is essential to prevent discrimination and promote equitable outcomes, particularly as AI is increasingly used in high-stakes decision-making. AI systems, if not properly managed, can inadvertently reinforce societal biases present in their training data, leading to discriminatory practices in sectors like hiring, finance, and criminal justice. (Source)
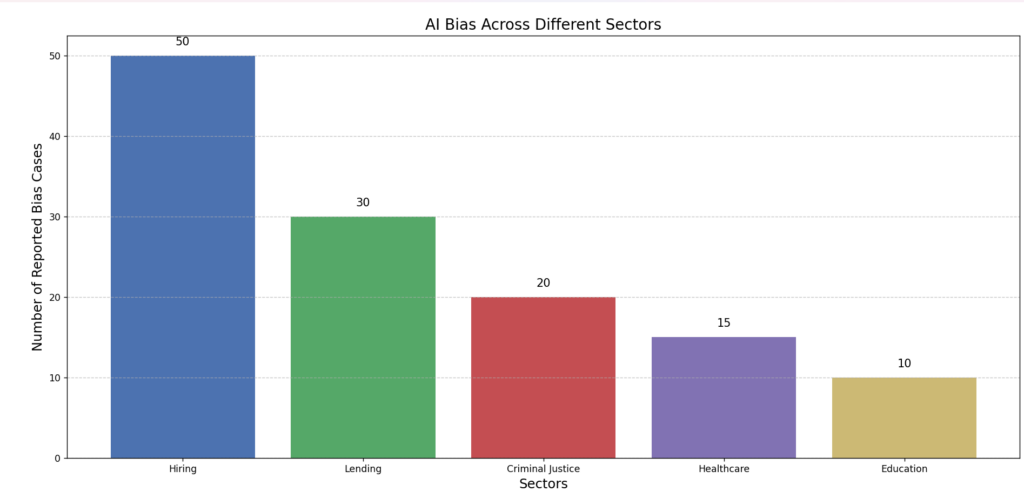
Note: illustrative examples intended to demonstrate how AI bias across different sectors
Examples of Bias in AI Systems
- Hiring Platforms: AI-driven hiring systems trained on biased historical data may favor certain demographics over others, unintentionally reinforcing pre-existing inequalities. This creates an unfair hiring process that can exclude qualified candidates from underrepresented backgrounds.
- Lending Applications: In financial services, lending applications assessing creditworthiness based on biased datasets may unfairly limit financial opportunities for specific groups. This creates a risk of discriminatory lending practices, reducing access to resources for underserved populations.
Impact of Bias: These biases can erode public trust in AI systems and result in significant regulatory and reputational risks for organizations, potentially affecting compliance and brand image.
Real-world examples of AI Bias
Sector | Incident / Study | Details / Percentages |
---|---|---|
Hiring | Amazon’s AI Recruiting Tool (2018) | Biased against female candidates; specific percentages not disclosed. |
HireVue’s AI Assessments (2020) | Women scored 7% lower; certain ethnic groups scored up to 10% lower. | |
Pymetrics’ AI for Hiring (2019) | Age bias favoring 30-40 age group by 12%; educational bias favoring top-tier universities by 15%. | |
Lending | Apple Card Credit Limits (2019) | Women received credit limits 20% lower than men with similar profiles. |
LendingClub’s AI Lending Models (2021) | Minority applicants faced 10% higher rejection rates compared to white applicants. | |
ZestFinance’s Credit Scoring Algorithm | African American borrowers had 7% lower approval rates compared to white borrowers. |
Best Practices for Mitigating Bias
To address bias, organizations should implement the following practices:
- Diverse Training Data: Use datasets that represent the diversity of the population served by the AI system. This approach reduces the risk of biased outcomes by providing the model with a well-rounded view of different demographics.
- Regular Model Audits: Conduct frequent audits to identify potential bias in the AI system. Regular reviews help ensure that any emerging biases are caught and corrected promptly.
- Utilize Bias Detection Tools: Leverage tools like IBM’s AI Fairness 360 to detect and address bias during model development. These tools support developers in building fairer and more inclusive models.
Sustainability and Compliance: By establishing these practices, organizations not only align with ethical AI standards but also promote long-term sustainability and fairness in AI applications, fostering inclusivity across all user groups.
Key Ethical Principles in AI Development
To create responsible and trustworthy AI systems, organizations must incorporate fundamental ethical principles across AI processes. By focusing on fairness, transparency, privacy, and accountability, decision-makers can enhance the credibility, safety, and social acceptance of their AI technologies, while also navigating regulatory requirements and managing risks.
Fairness and Bias Mitigation
Fairness in AI is essential for preventing discrimination and promoting equitable outcomes, particularly in automated decision-making where bias can unintentionally emerge from historical data. Bias in AI algorithms can perpetuate inequalities, affecting marginalized groups and creating ethical and legal risks for organizations.

Real-World Example | Challenges and Impact |
---|---|
Hiring Platforms | Bias in hiring algorithms may favor certain demographics, creating discriminatory hiring practices and harming organizational diversity efforts. |
Lending Applications | Bias in credit scoring can restrict access to financial resources, disproportionately affecting low-income or minority groups. |
Best Practices for Bias Mitigation
To address these risks, organizations can adopt several measures:
- Use Diverse Training Data: Ensure datasets represent a wide range of demographic and cultural groups.
- Conduct Regular Bias Audits: Periodic checks can help detect and reduce emerging biases in AI models.
- Implement Fairness Tools: Leverage tools like IBM’s AI Fairness 360, which identifies and mitigates bias in AI algorithms.
- Engage External Reviewers: Independent audits can provide an unbiased perspective, further enhancing fairness.
Transparency and Explainability
Transparency is essential in building trust in AI systems, making decision-making processes accessible and understandable to both users and regulators. Explainable AI not only enhances public trust but also ensures organizations meet compliance requirements.
Use Case
Under the European Union’s AI Act, transparency is mandated for high-risk applications like credit scoring, ensuring individuals and institutions can comprehend how AI-derived decisions are made. Lack of explainability in such cases could lead to compliance issues, undermining both regulatory adherence and stakeholder trust.
Implementation Tips for Explainability
- Employ Explainable AI Models: Use interpretable models (e.g., decision trees, LIME) that provide insights into how decisions are reached. (Source)
- Simplify User Communication: Clear and concise language in model explanations aids understanding for non-technical stakeholders.
- Integrate Visual Tools: Graphs, flowcharts, or visual models can be used to represent decision-making pathways, improving accessibility and trust.
- Assess Explainability Standards: Regular reviews ensure compliance with frameworks like the EU’s AI Act.
Privacy and Data Protection
Safeguarding personal and sensitive data is a core ethical obligation in AI, especially in applications that handle large volumes of identifiable information. Failure to ensure data protection can lead to significant privacy breaches, regulatory penalties, and a loss of user trust.
Example | Privacy Risks |
---|---|
Facial Recognition | This technology involves capturing and storing sensitive biometric data, raising concerns about data misuse and surveillance. |
Implementation Strategies for Data Protection
- Adhere to GDPR Standards: Compliance with regulations like GDPR is critical for protecting user data, particularly within the EU. (Source)
- Use Anonymization Techniques: De-identifying data reduces privacy risks and limits exposure of sensitive information.
- Limit Data Access: Implement access control measures to restrict who can view or use personal data, strengthening internal security.
- Conduct Privacy Impact Assessments: Assessments help identify risks before launching new AI applications, ensuring data protection measures are effective.
Accountability
Assigning accountability in AI is crucial for handling outcomes and resolving issues when things go wrong. This is particularly important in complex AI applications, where decisions are made autonomously. Accountability ensures organizations remain responsible for their systems, regardless of the complexity of AI decisions. (Source)
Illustration | Complex Accountability Layers |
---|---|
Autonomous Vehicles | In cases of accidents involving autonomous cars, accountability must be clearly established, covering manufacturers, developers, and users. |
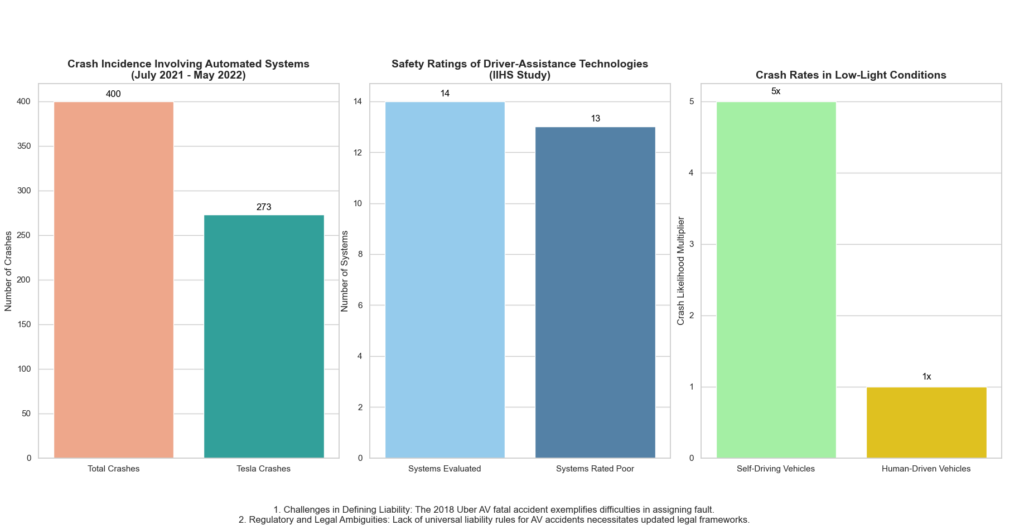
Sources:
- https://www.nhtsa.gov/press-releases/initial-data-release-advanced-vehicle-technologies
- https://link.springer.com/article/10.1007/s13198-020-00978-9
- https://www.statista.com/chart/32985/collisions-crashes-per-motor-vehicle-vehicle-miles-traveled-by-type-of-vehicle/
- https://www.brookings.edu/articles/the-evolving-safety-and-policy-challenges-of-self-driving-cars/
- https://www.abc.net.au/news/2024-06-19/self-driving-cars-report/103992024
Practical Steps for Ensuring Accountability
- Establish Oversight Mechanisms: Form ethics committees or assign designated teams to oversee AI practices and enforce accountability.
- Set Clear Guidelines: Define responsibilities across all levels of AI development and usage, from data management to deployment.
- Implement Incident Reporting Protocols: Develop processes to document and address errors or failures, ensuring issues are handled promptly and transparently.
- Review Accountability Policies Regularly: Update guidelines as new AI uses emerge, maintaining compliance with evolving regulations and standards.
Implementing Ethical AI Frameworks in Organizations
To maintain responsible AI development and usage, organizations must embed ethical principles into every stage of the AI lifecycle. Decision-makers play a crucial role in fostering a culture of ethical awareness, collaboration, and compliance. This section outlines key practices for organizations to effectively integrate ethical AI frameworks.
Developing Ethical Guidelines
Creating comprehensive ethical guidelines is fundamental to setting clear standards for AI development and deployment. These policies should address fairness, transparency, privacy, and accountability throughout the AI lifecycle, ensuring that ethical considerations are embedded in all processes.
Actionable Steps
- Establish Core Ethical Policies: Draft policies that define acceptable AI use cases and standards for data handling.
- Utilize Established Frameworks: ISO guidelines and AI ethics toolkits provide a solid foundation, helping organizations align with industry-recognized standards.
- Adapt Guidelines to Context: Tailor policies to specific applications and regulatory requirements, ensuring relevancy across various AI projects.
Collaboration Across Disciplines
AI ethics requires diverse perspectives to ensure comprehensive oversight and balanced decision-making. By incorporating viewpoints from technology, ethics, law, and social science, organizations can address potential ethical gaps.
Recommended Approach
- Engage Experts Across Fields: Form collaborative teams that include ethicists, legal advisors, and technologists.
- Consult External Specialists: Use third-party experts for additional perspectives and independent audits, which add credibility and neutrality.
- Foster Cross-Functional Communication: Encourage regular inter-departmental discussions to ensure that ethical considerations are integrated throughout AI development and application.
Investing in Continuous Education
Continuous training on ethical AI practices keeps teams informed and adaptable in a rapidly evolving landscape. By prioritizing education, organizations can maintain a knowledgeable workforce that upholds ethical standards.
Implementation
- Conduct Regular Workshops: Host sessions on current ethical practices, legal requirements, and emerging AI standards.
- Offer Certifications: Support employees in pursuing certifications in responsible AI and data ethics to bolster internal expertise.
- Implement Knowledge-Sharing Initiatives: Create an internal repository for best practices, case studies, and regulatory updates, accessible across departments.
Establishing Oversight Mechanisms
Effective oversight ensures that AI systems remain compliant and ethically sound. Ethics boards or committees can monitor AI projects, providing guidance and addressing concerns as they arise.
Oversight Models | Purpose and Examples |
---|---|
Ethics Committees | Facilitate ongoing ethical evaluations, as seen in organizations like Google. |
Independent Review Boards | External reviews provide impartial assessments, helping to identify potential biases. |
Internal Compliance Teams | In-house teams dedicated to AI ethics ensure adherence to guidelines and policies. |
Role of Oversight
Establishing dedicated ethics boards provides ongoing assessment and adjustment of AI practices. These committees should have authority to enforce guidelines, review AI projects, and recommend changes where needed. Through consistent monitoring and transparent accountability, organizations can better navigate the complexities of ethical AI use.
Global Initiatives and Regulations Impacting AI Ethics
The global regulatory landscape for AI ethics is evolving rapidly, with key initiatives aiming to standardize responsible AI practices worldwide. Decision-makers need to stay informed of these developments to ensure compliance and leverage emerging standards that support privacy, transparency, and accountability.
Regulatory Developments
Several regions have introduced significant AI regulations, setting benchmarks for ethical AI governance.
- European Union’s AI Act: The EU’s AI Act is a landmark regulation targeting high-risk AI applications like credit scoring, healthcare, and law enforcement. It mandates transparency, risk assessment, and bias mitigation, setting strict requirements that influence global AI practices.
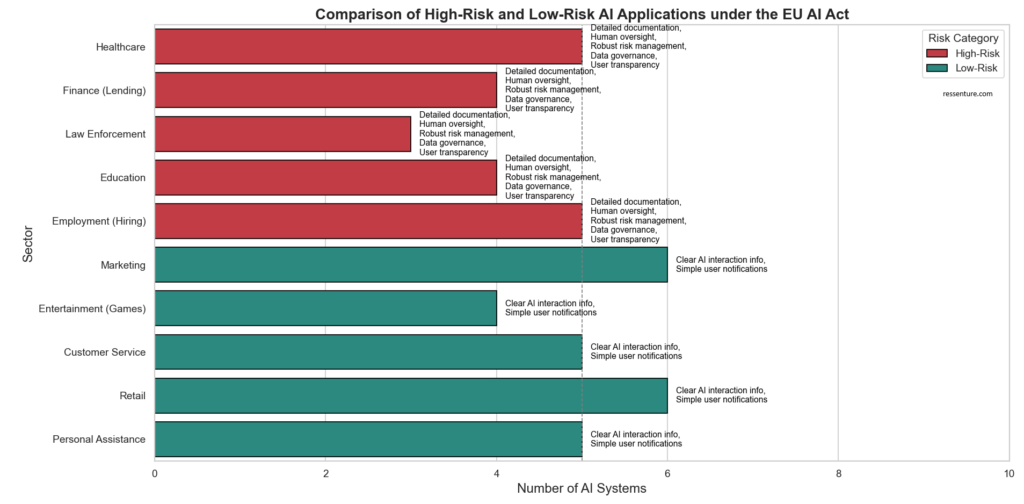
- Comparative Overview: While the EU leads with stringent regulations, the U.S. is adopting a sector-specific approach, with guidelines from the FTC focusing on transparency and data privacy. In contrast, China has introduced data security laws emphasizing control and state oversight.
Region | Focus | Enforcement Rigor |
---|---|---|
EU | High-risk applications, transparency | Strict |
U.S. | Sector-specific, privacy-focused | Moderate |
China | Data security and control | High |
Implications
These varying regulatory approaches underscore the need for adaptable, globally compliant AI frameworks, particularly for organizations operating across multiple jurisdictions. (Source)
Standards and Best Practices
International bodies have established guidelines that serve as best practices for ethical AI development, influencing industry norms.
- ISO Standards: The ISO offers guidelines on AI risk management, transparency, and data governance, aiding organizations in adopting compliant practices.
- IEEE Ethics Guidelines: IEEE’s ethical standards provide actionable steps for transparency, bias prevention, and accountability, promoting responsible AI use.
- OECD’s Ethical AI Framework: The OECD focuses on fairness and accountability, encouraging practices that align AI development with public values.
Influence on Organizations
These standards help organizations implement best practices across privacy, transparency, and accountability, setting a baseline for ethical compliance.
Future of AI Ethics Regulation
The AI regulatory landscape is expected to intensify, with increasing emphasis on data protection, transparency, and accountability.
- Trend Analysis: Regulations are likely to expand to include broader transparency requirements and stricter data privacy mandates. There may also be increased penalties for non-compliance as regulators aim to hold organizations accountable for ethical lapses.
- Impact for Decision-Makers: Decision-makers should anticipate evolving compliance requirements and prepare by integrating flexible AI frameworks and staying updated on international regulatory trends. Early adoption of privacy and transparency practices will position organizations advantageously as regulations intensify.
Balancing Innovation with Responsibility: Best Practices and Strategies
Balancing AI innovation with ethical responsibility is essential to harness AI’s benefits while maintaining public trust. Organizations can implement targeted strategies to foster responsible innovation, build stakeholder trust, and ensure inclusivity and fairness in AI development. These best practices offer guidance for decision-makers on integrating ethical considerations into AI initiatives.
Encouraging Responsible Innovation
Creating an environment that supports both innovation and ethical responsibility enables sustainable AI growth. Decision-makers should prioritize ethical frameworks that guide teams in aligning technological advancements with societal values.
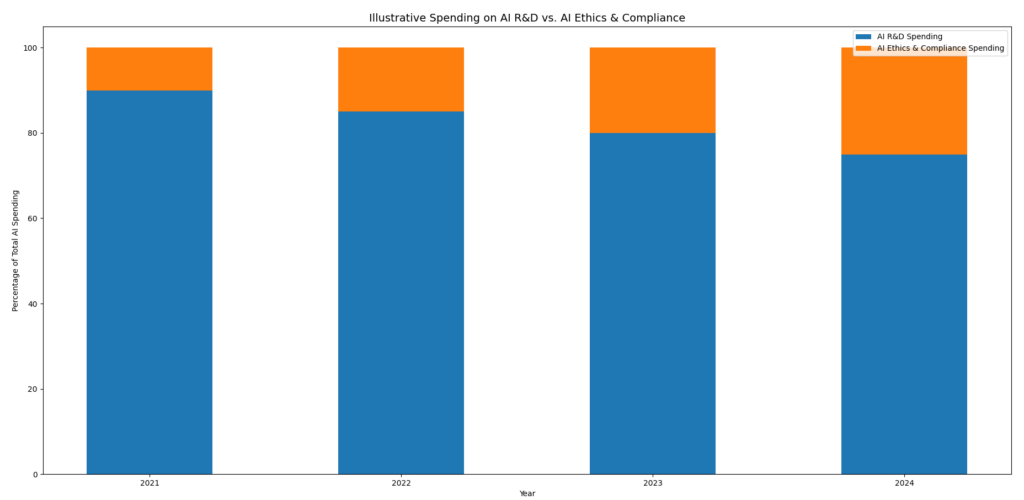
Source:
- Statista (2021): Reports that 89% of industrial manufacturing organizations have implemented ethics policies for AI. This suggests a growing focus on ethical AI in the industrial sector.
- AI Index Report (2024): Notes that the U.S. federal budget for AI R&D increased from $1.7 billion in 2022 to $1.9 billion in 2024, indicating sustained investment in AI R&D.
- Stanford HAI (2023): Reports that legislative bodies in 127 countries passed 123 AI-related bills, with a focus on ethical considerations, highlighting an increase in regulatory attention on AI ethics.
- SpringerLink (2024): Emphasizes the need for objective metrics in ethical AI development, indicating that while ethics awareness is growing, measurable standards are still emerging.
Tips for Implementation:
- Establish Ethical Innovation Frameworks: Create policies that mandate ethical reviews at each stage of AI development.
- Conduct Regular Audits: Schedule routine audits to monitor adherence to ethical standards, adjusting as needed to address emerging risks.
- Set Measurable Goals: Define clear objectives for responsible AI, allowing teams to balance innovation with accountability.
Engaging Public and Stakeholder Trust
Public and stakeholder trust is vital for successful AI adoption. Transparency and accountability are key to fostering trust, as they demonstrate an organization’s commitment to responsible AI practices.
Strategies for Building Trust:
- Transparent Communication: Regularly disclose AI’s intended use, limitations, and potential impacts to users and stakeholders.
- Host Public Forums: Engage communities and stakeholders through forums or webinars that address concerns and gather feedback.
- Implement Clear Data Usage Policies: Publish detailed data policies that outline how data is collected, processed, and protected.
Promoting Inclusive and Fair AI Systems
For AI to serve diverse populations effectively, inclusivity and fairness must be embedded into its design and deployment.
Best Practices for Inclusivity | Description |
---|---|
Diverse User Testing | Involve individuals from different demographic backgrounds to ensure the AI meets varied needs. |
Continuous Bias Monitoring | Regularly monitor AI outputs for bias, refining models as necessary to uphold fairness. |
Inclusive Design Standards | Adopt guidelines that ensure AI tools are accessible to all user groups. |
Practical Steps for Decision-Makers
Decision-makers play a critical role in setting ethical standards and leading by example. Specific actions can help ensure that AI systems align with organizational values and ethical goals.
Actionable Insights:
- For C-Level Executives: Lead by establishing a top-down commitment to ethical AI, incorporating it into the corporate mission.
- For Data Officers: Ensure all data sources are vetted for bias and compliance, aligning data management practices with ethical standards.
- For Compliance Managers: Conduct regular ethics assessments and provide training on regulatory changes to keep teams informed.
FAQs on Ethical AI Frameworks
Here are some most asked questions asked around the topic.
What are the core ethical principles in AI?
Core principles include fairness, transparency, privacy, and accountability to guide responsible AI use.
How can organizations ensure transparency in AI decision-making?
By using explainable models and clear communication, organizations can make AI decisions understandable to users.
Why is accountability important in AI ethics?
Accountability ensures that organizations are responsible for AI outcomes, promoting trust and managing risks.
What is the role of global regulations like the EU AI Act in shaping AI ethics?
Regulations like the EU AI Act set standards for high-risk AI applications, driving compliance and ethical practices.
How can AI bias be effectively mitigated?
Bias can be reduced by using diverse datasets, conducting regular audits, and employing fairness tools.
Conclusion
Balancing innovation with responsibility is essential for the sustainable development and deployment of AI. As AI becomes increasingly integrated into critical areas of society, prioritizing ethical frameworks that promote fairness, transparency, privacy, and accountability safeguards public trust and aligns AI systems with societal values. By embedding these principles into every stage of AI development, organizations can leverage AI’s transformative potential responsibly, ensuring benefits without unintended harm.
For leaders, adopting proactive ethical frameworks isn’t just a regulatory requirement—it’s a strategic advantage. Embracing responsible AI practices enables organizations to harness AI’s potential effectively, build lasting stakeholder trust, and position themselves as champions of responsible innovation in a rapidly evolving landscape.